<Show, Attend and Tell: Neural Image Caption Generation with Visual Attention>
1. This paper brings about "hard attention". The authors introduce two attention-based image caption generators under a common framework: 1) a "soft" deterministic attention mechanism trainable by standard back-propagation methods and 2) a "hard" stochastic attention mechanism trainable by maximizing an approximate variational lower bound or equivalently by REINFORCE.
2. We first introduce their common framework. The model takes a single raw image and generates a caption y encoded as a sequence of 1-of-K encoded words.

where K is the size of the vocabulary and C is the length of the caption.
3. We use a CNN to extract a set of feature vectors which we call annotation vectors.

4. We use LSTM to produce a caption by generating one word at every time step conditioned on a context vector, the previous hidden state and the previously generated words. The structure is like that:
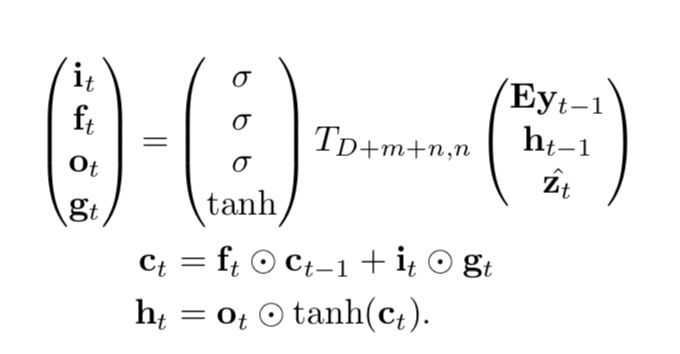
where is a simple affine transformation with parameters that are learned.
The diagram is like that:
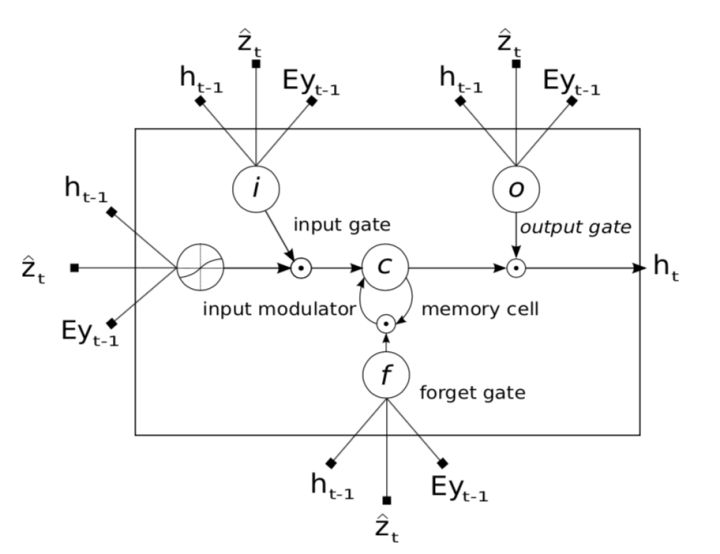
5. In simple terms, the context vector is a dynamic representation of the relevant part of the image input at time t. We define a mechanism
that computes
from the annotation vectors
, I=1,...,L corresponding to the features extracted at different image locations. For each location i, the mechanism generates a positive weight
which is the relative importance of it. The
is computed by that:
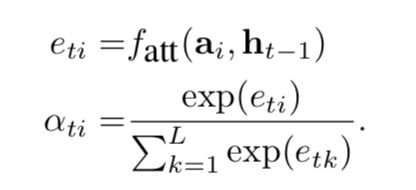
where a is computed by a multilayer perceptron.
6. Once the weights are computed, the context vector is computed by
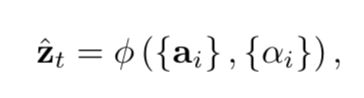
where is a function that returns a single vector given the set of annotation vectors and their corresponding weights.
7. The initial memory state and hidden state of the LSTM are predicted by an average of the annotation vectors fed through two separate MLPs (init, c and init, h):
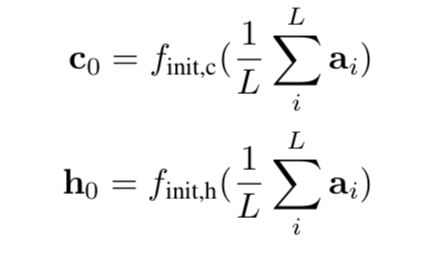
8. We use a deep output layer to compute the output word probability given the LSTM state, the context vector and the previous word:

9. Stochastic "Hard" Attention.
We define a local variable as where the model decides to focus attention when generating the
word.
is an indicator one-hot variable representing that i-th location (out of L) is the one used to extract visual features. We can assign a multinoulli distribution parametrized by
, and view
as a random variable:
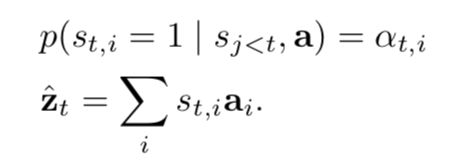
We define a new objective function that is a variational lower bound on the marginal log-likelihood
of observing the sequence of words
given image features
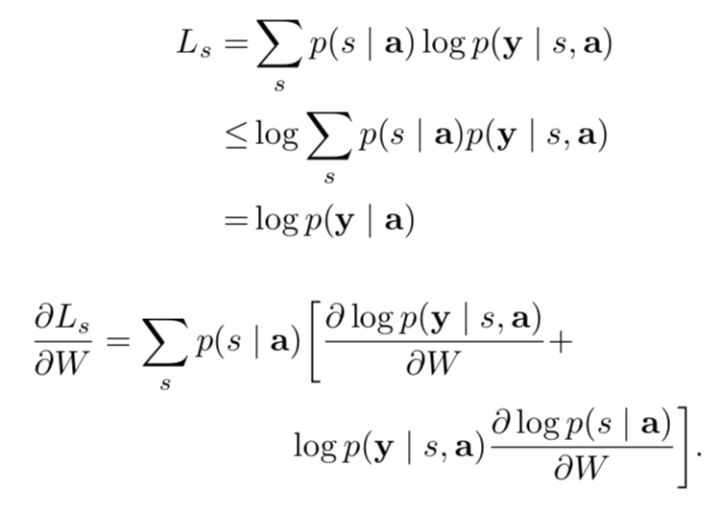
(I think that there is something wrong with the formula... I use the [f(x)g(x)]'=f(x)'g(x) + f(x)g(x)' to conduct it but failed...)
The equation above suggests a Monte Carlo based sampling approximation of the gradient with respect to the model parameters. This can be done by sampling the location from the distribution above, which is:
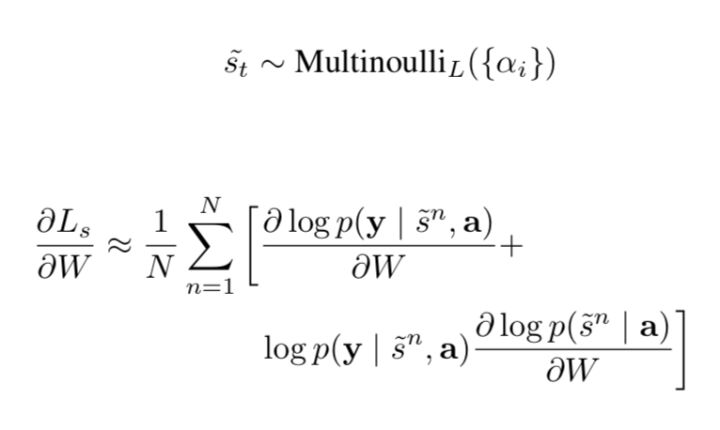
A moving average baseline is used to reduce the variance in the Monte Carlo estimator of the gradient. Upon seeing the mini-batch, the moving average baseline is estimated as an accumulated sum of the previous log likelihoods with exponential decay:

To further reduce the estimator variance, an entropy term on the multinouilli distribution H[s] is added. We set the sampled attention location to its expected value
.
Both techniques improve the robustness of the stochastic attention learning algorithm.
The final rule is like that:
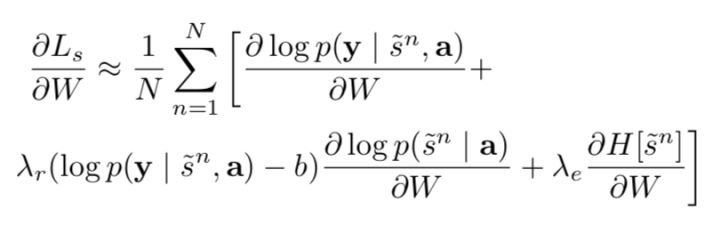
10. Deterministic "Soft" Attention
Learning stochastic attention requires sampling the attention location each time, instead we can take the expectation of the context vector directly,
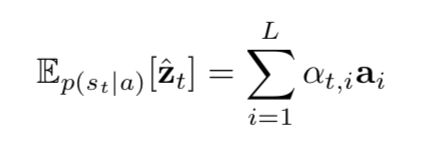
and formulate a deterministic attention model by computing a soft attention weighted annotation vector:

The whole model is smooth and differentiable under the deterministic attention. So learning end-to-end is trivial by using standard back-propagation.
11. We define the NWGM(normalized weighted geometric mean for the softmax word prediction:
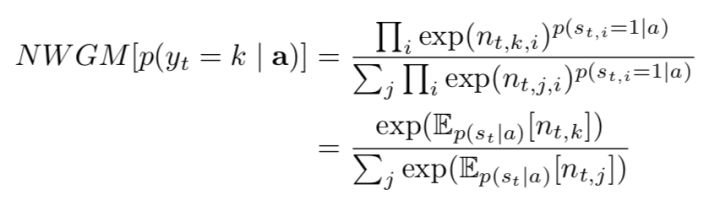
12. The model is trained end-to=end by minimizing the following penalized negative log-likelihood:
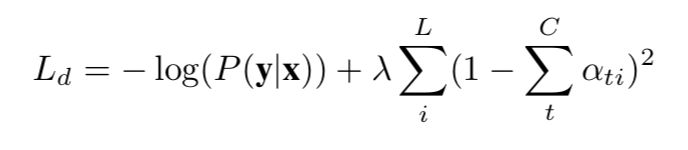
which encourage , and it is called DOUBLY STOCHASTIC ATTENTION.